Özyeğin Üniversitesi, Çekmeköy Kampüsü Nişantepe Mahallesi Orman Sokak 34794 Çekmeköy İstanbul
Telefon : +90 (216) 564 90 00
Fax : +90 (216) 564 99 99
info@ozyegin.edu.tr
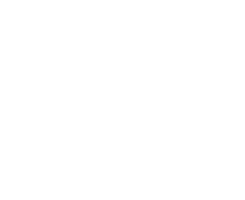
Thesis Defense - Ömer Fatih Dokumacı (MSPHYS)
Ömer Fatih Dokumacı – M.Sc. in Physics
Prof. Mehmet Burçin Ünlü – Advisor
Prof. Bora Işıldak – Co-advisor
Date: 02.08.2024
Time: 11.00
Location: AB1 245
“Jet Energy Corrections with Deep Learning”
Prof. Mehmet Burçin Ünlü, Özyeğin University
Prof. Bora Işıldak, Yıldız Technical University
Prof. Taylan Akdoğan, Özyeğin University
Prof. H. Fatih Uğurdağ, Özyeğin University
Ass. Prof. Hale Sert, İstanbul University
Abstract:
The LHC at CERN accelerates and collides protons at enormous energies and currently is the most powerful particle accelerator available. The CMS detector is a sophisticated device designed to record the cascade of particles arising from these collisions. With the aid of Monte Carlo simulations, detected particles are reconstructed and clustered together to create physics objects known as jets. The importance of jets is linked to the indirect study of quarks and gluons, since they are not observed freely in nature. Tasks such as reconstruction of high-energy processes, identification of particle interactions and search for new physics beyond the Standard Model all rely on precise study of jets. Due to the detector response and additional factors, the measured energies of the jets need to be calibrated according to simulated truth values. Hence, in the CMS experiment, a sequence of jet energy correction methods is applied to align the jet energies with the true values. In this thesis, we utilized a deep learning approach to further improve standard jet corrections. In recent years, the incorporation of machine learning algorithms has shown great results in high-energy physics studies. Tasks such as jet tagging and particle reconstruction have benefited from recent developments in deep learning. For the jet energy correction task, which is a regression problem, we used two models: the Deep Sets-based Particle Flow Network and a more straightforward fully connected deep neural network. The results of the corrections are presented primarily by two metrics that allow for a comprehensive understanding of performance: average response and relative jet energy resolution. The models surpassed standard energy corrections on a large scale in both metrics.
Bio:
Ömer Fatih Dokumacı received his B.Sc degree in Physics from İstanbul University. He is currently pursuing an M.Sc in Physics at Özyeğin University and his research interests are particle physics and computational physics.