Özyeğin Üniversitesi, Çekmeköy Kampüsü Nişantepe Mahallesi Orman Sokak 34794 Çekmeköy İstanbul
Telefon : +90 (216) 564 90 00
Fax : +90 (216) 564 99 99
info@ozyegin.edu.tr
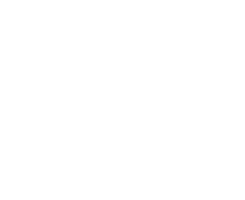
Thesis Defense - Sami Menteş (MSCS)
Sami Menteş – M.Sc. Computer Science
Asst. Prof. M. Furkan Kıraç – Advisor
Date: 26.05.2022
Time: 13:00
Location: AB1 412
“Adapting Bilateral Networks to Monocular Depth Estimation for Real-time Inference”
Thesis Committe
Asst. Prof. M. Furkan Kıraç, Özyeğin University
Asst. Prof. Reyhan Aydoğan, Özyeğin University
Asst. Prof. Berk Gökberk, Boğaziçi University
Abstract:
Monocular Depth Estimation (MDE) is an important computer vision application area for many industry-related advances. Due to its deployment needs, the inference time of the depth estimation algorithm also plays a crucial role among other accuracy metrics. With the recent advances in the usage of Convolutional Neural Networks (CNNs) on other time-constrained computer vision tasks, many efficient feature extractors have been studied and adopted from MDE models as the backbone. Although those feature extractors have shown great improvement in throughput, the popular encoder-decoder architecture used by Real-time MDE models also relies on a decoder network for upsampling. Following a similar approach, stacking multi-channel convolutional layers on a decoder significantly hinders the inference time. In this study, we investigate the use of Bilateral Networks in Real-time MDE tasks. To achieve this, we first manipulate a recently introduced real-time segmentation model (STDC-Seg) for the MDE problem. Once we achieve the real-time inference speed, we tailor the backbone structure and attention modules of the network with respect to the needs of MDE for further improving prediction accuracy. Finally, we train the models on a well-known KITTI dataset and compare our results with the models of KITTI Eigen Split MDE Benchmark and also with the previous real-time models. Our experimental results show that our real-time method achieves on par metric performance with the state-of-the-art models which are not subject to any time-constraint.
Bio:
Sami Menteş graduated from Sainte Pulcherie French High School in 2015. He received his B.Sc. degree in Computer Science from Özyeğin University in June 2019. He has been pursuing his M.Sc. degree also in Computer Science at Özyeğin University since September 2019, under the supervision of Asst. Prof. M. Furkan Kıraç. His research interests mainly involve applications of Deep Learning in Computer Vision related problems.